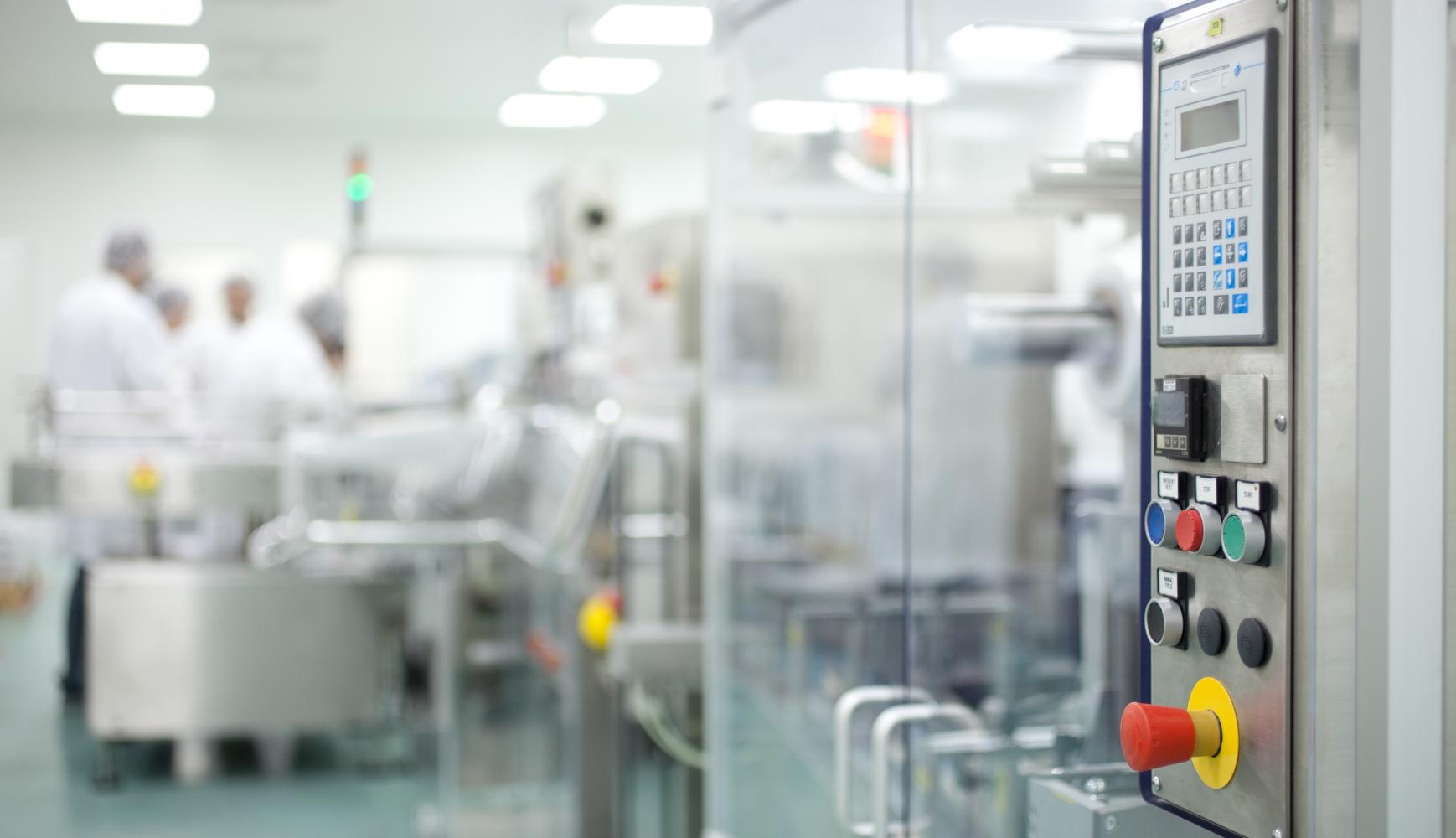
Quality over Quantity
With every passing year we at Saber Informatics are seeing increasing demand for managing the quality (not quantity) of data generated and collected in preclinical life science labs and in clinical research.
While it is a commonly repeated maxim that "big data" is changing the way we work, attention to data quality in small but complex datasets ensures the continuity of pharmaceutical research. Without attention to quality there is no confidence in results, therefore no regulatory approval. This explains the persistent focus on "small data" in the industry rather than on the big.
The terms "data lake" and such may sound inspiring in theory but automated data clean-up or indexing in practice are not really effective without manual curation, as crucial annotations and data links have already been lost. For that reason any successful "data lake" project must include a healthy dose of professional services from the vendor. Beware. The real data cleanup will happen on billable time.
A Little Focus Goes a Long Way
Recognizing that cleaning up afterwards is a lot more resource-expensive than keeping the data in good shape at all times, some of the companies in the industry have put quality processes in place for dealing with R&D data. The gist of which is "authorize access, document algorithms, keep an audit trail of changes". I would also add to that "keep the original source data archived". It's not that hard to do if done early on.
A little focus on quality early on goes a long way towards keeping everything in order and accounted for. Here are a couple of examples from recent Saber Informatics projects.
-
A screening biology lab runs assays the results of which are registered into corporate databases so that co-located chemists and also the rest of the company can use them in ongoing projects. From time to time data scientists in research IT and on the chemistry side have questions about a data point or a result linked to a compound lot. Such as "why is there a duplicate" or "why is that missing, I was just told the experiment was run weeks ago". It turns out that having a reasonably solid audit trail of when,who,how registered the data helps answer such questions in minutes. Sometimes it's user error where an experiment was registered with the wrong instrument data file, sometimes input data contained typos, etc. The most important outcome of having a data audit trail is that everyone involved is confident that experimental results won't "fall through the cracks" and everything is accounted for.
-
A pharma runs clinical trials at a large number of sites simultaneously. There are large numbers of containers with investigational drugs shipped between company warehouses and trial sites. Sites sometimes do make mistakes and record an incorrect event date, container ID, or omit a record altogether. Working collaboratively with the Client's clinical operations team, Saber Informatics implemented a process that runs each Excel file through a documented set of data validation checks designed to flag any data inconsistencies. As a result the Client has measurably more confidence in tracking each container from creation to destruction (as required by federal and state authorities) and is much better prepared for regulatory inspections.
For the foreseeable future, attention to data quality as opposed to quantity will continue to be the key to the continuity of pharmaceutical R&D.